
Proteome-scale quantitative affinity-purification-followed-by-MS (AP/MS)
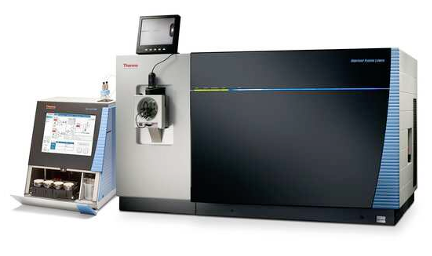
All proteins function through interacting with other biomolecules, especially other proteins. We are highly experienced with advanced, quantitative AP/MS techniques to accurately assess the protein interaction network and how they change by disease mutations and under different conditions on the whole proteome scale. Our Orbitrap Fusion Lumos Tribrid Mass Spectrometer is a state-of-the-art instrument capable of SPS-MS3 which provides highly sensitive and accurate analysis of TMT-labeled complex biological samples. We are also proficient in other labeling and non-labeling techniques which we regularly use when appropriate. These technological advancements have expanded the scope of proteomic research, enabling the identification of potential biomarkers for disease diagnosis and monitoring disease progression, but are also proficient in helping interrogate basic research questions.
Relative Publication: Zhou et al. Nature Biotechnology, 2023
Next-generation interactome mapping using MS-cleavable cross-linking MS (XL-MS)
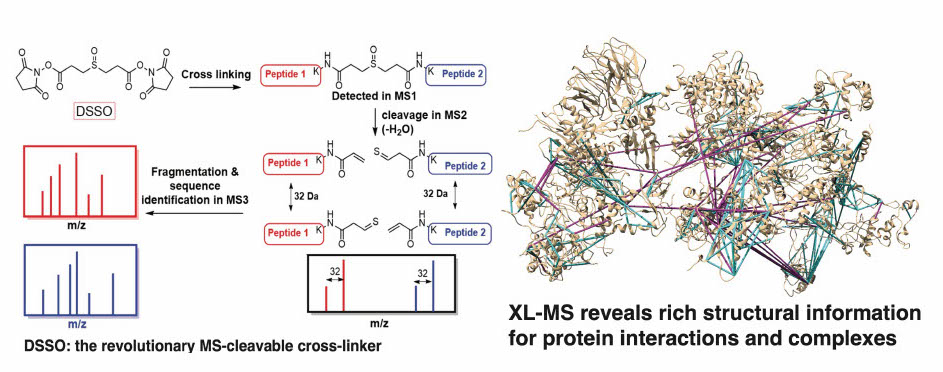
In recent years, major breakthroughs in chemistry and instrumentation have introduced major advancements in cross-linking MS. We have developed a revolutionary MS3-centric search engine, MaXLinker, for XL-MS using novel MS-cleavable crosslinkers. Working closely with Hening Lin’s lab, we are developing the next-generation of interactome mapping technologies to generate patient/tissue-specific interactome networks with structural details for large cohorts, which is simply not possible with current technologies.
Relative Publication: Yugandhar et al. MCP, 2018; Yugandhar et al. Nature Methods, 2020
High-resolution quantitative mapping of sub-cellular proteomes (Proximity-labeling MS: APEX-MS, TurboID, mini-TurboID, Split-TurboID, CAPTURE 2.0 (dCas9-BirA), C-BERST (dCas9-APEX2))
Quantitative proximity-labeling MS is a powerful technique for identifying organelle-specific interactomes/proteomes and their dynamics. By labeling proteins with biotin, it is possible to purify them efficiently using streptavidin-based tools allowing for the detection of low-abundance, transient, and specifically expressed proteins both in vivo and in vitro. We have implemented (or are developing) several cutting-edge technologies that allow us to target specific proteins (APEX-MS2 and TurboID) or target specific genomic loci [CAPTURE 2.0 (dCas9-BirA) and C-BERST (dCas9-APEX2)].INtegrated prOtein INteractome perTurbation screening (InPOINT) pipeline
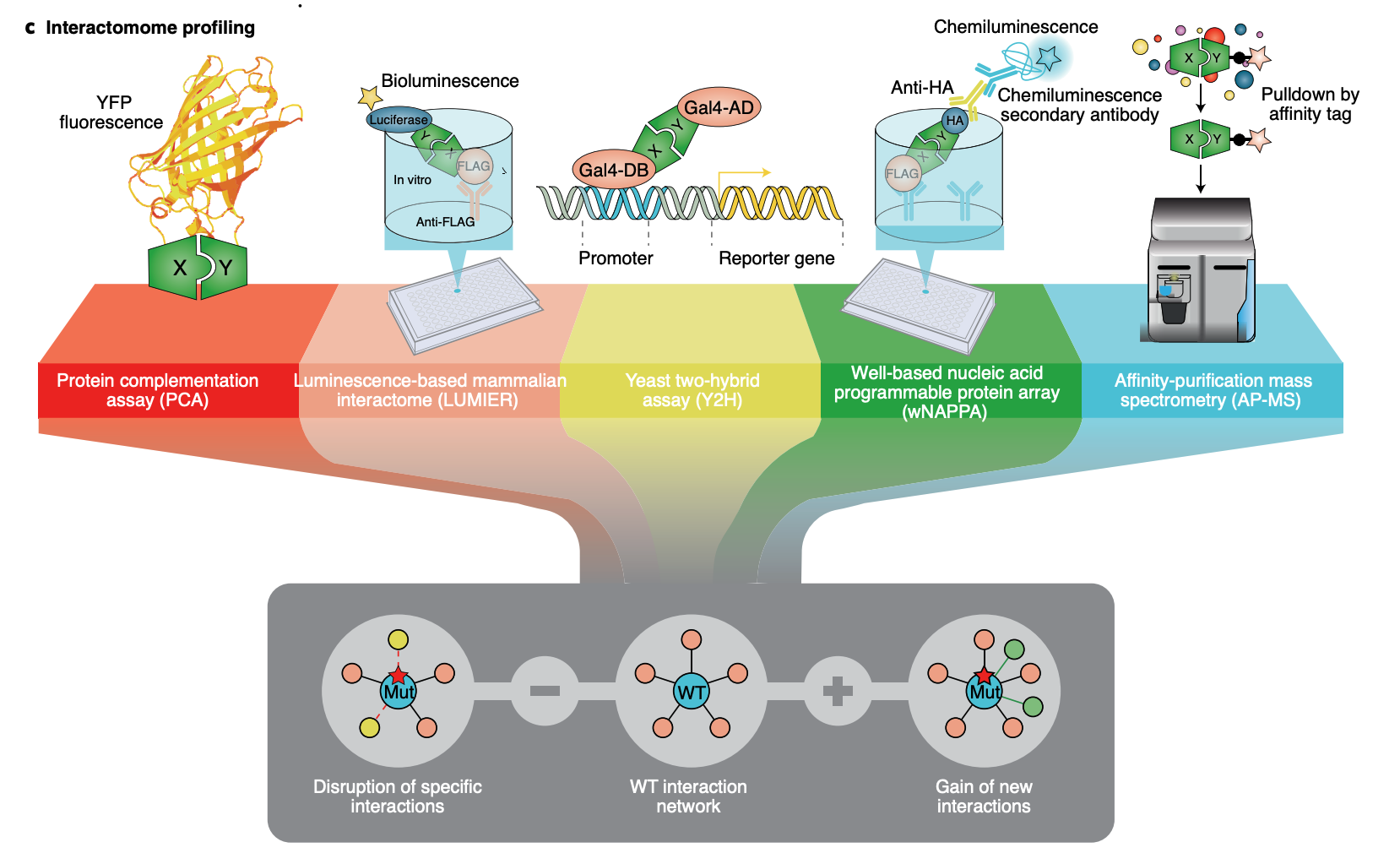
Identification of functional genetic variants based solely on sequence information is extremely challenging. Instead, the effects of coding variants can be successfully investigated in the context of protein-protein interactome networks. Our InPOINT pipeline is a powerful, high-throughput pipeline that utilizes 5 orthogonal protein interaction assays to quantify the effects of coding variants on protein stability and protein-protein interactions. The combination of multiple assays ensures the quality of the data and nearly eliminates false-positive results.
Relative Publication: Chen et al. Nature Genetics, 2018; Fragoza et al. Nature Communications, 2019
Single-cell proteomics with spatial resolution
Single-cell and spatial omics are revolutionizing biology; single-cell transcriptomics studies have clearly shown the heterogeneity of gene expression across cells and the importance of measurement at the single cell resolution. Proteins are the functional molecules in the cell, so it is naturally important to measure protein abundance and their changes at the whole proteome level across different cells with high spatial resolution (ideally at the single cell level). Complementing the existing antibody-based spatial/single-cell proteomics methods that employ imaging, barcoding, or mass spectrometry (e.g., CyTOF), obtaining a comprehensive view of the entire proteome at a high spatial resolution is crucial for uncovering novel factors that play a pivotal role in biological processes or diseases. With the recent development in mass spectrometry instrumentation (significantly increased sensitivity), multiplexing with tandem mass tags (TMT), Data-independent-acquisition (DIA) with deep neural network enabled search algorithms, and development of equipment for ultra low volume liquid and cell handing, we are developing innovative ultra-sensitive proteomics technologies with high spatial resolution.
Clone-seq
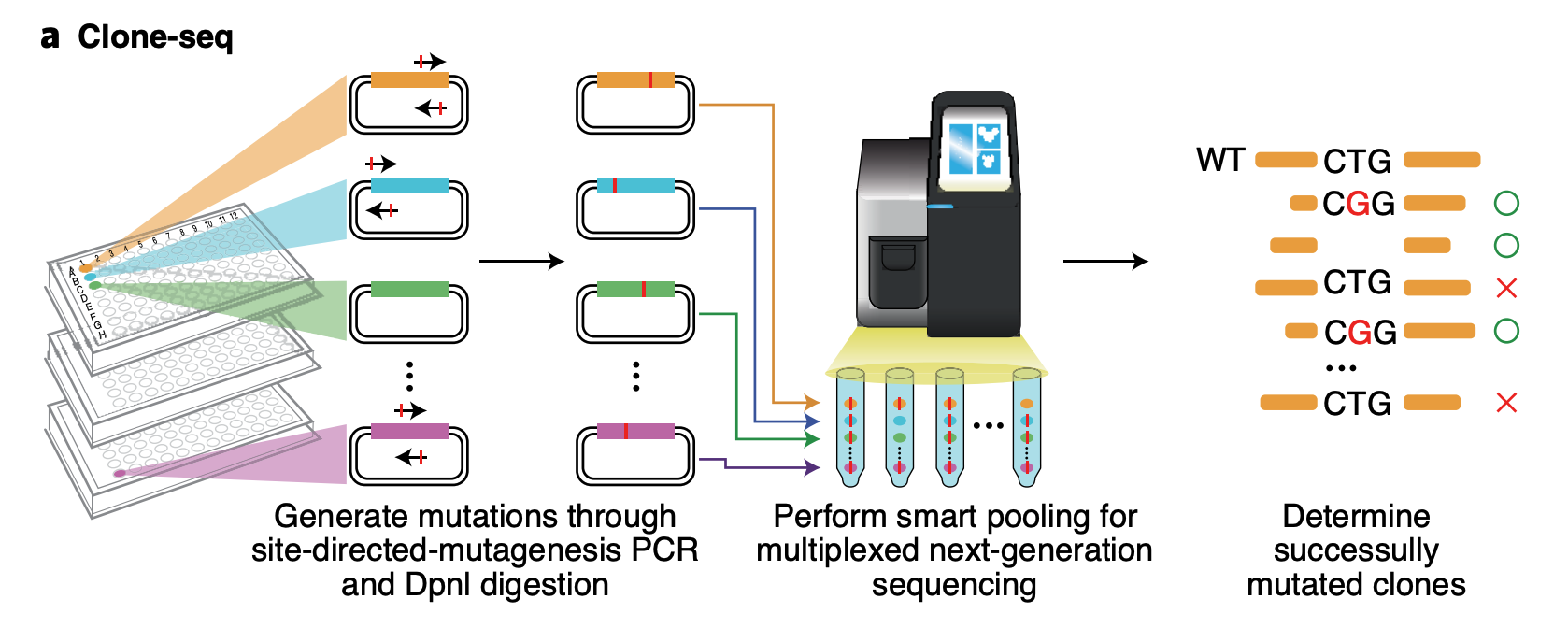
Clone-seq is a massively parallel site-directed mutagenesis approach that leverages next-generation sequencing to generate a large number of mutant alleles quickly and cost-effectively. We have successfully tens of thousands of gene (WT and mutant) and enhancer/promoter (WT and mutant) clones using Clone-seq, which allowed us to experimentally assay the functions of these elements and the impact of mutations.
Relative Publication: Fragoza et al. Nature Communications, 2019; Tippens et al. Nature Genetics, 2020
SOLARR-seq / eSTARR-seq
Classical STARR-seq assays require the cloning of candidate enhancers within the 3′-UTR of a reporter construct. We hypothesize that this cloning of exogenous sequences within the 3′-UTR could inadvertently lead to mRNA instability and thus confound enhancer activity readouts. To address this potential bias, we have modified our element STARR-seq assay such that the enhancer candidates are cloned downstream of the CPS and utilize element ID barcodes to assign activity scores to the enhancers. Using our refined assay, which we are terming Surveying OLigonucleotides via Active Regulatory Regions and sequencing (SOLARR-seq), we are re-testing previously published TRE libraries surveyed using our original eSTARR-seq, in addition to numerous new TRE collections designed to extract novel biological insight of enhancer units.PRO-cap and PRO-seq
PRO-cap, a nuclear run-on assay developed by John T. Lis' group, enables the identification of active transcriptional regulatory elements by mapping nascent transcription at transcription start sites (TSSs) at base pair resolution genome-wide. Moreover, by tracking nascent transcription, this methodology allows for the measurement of the dynamic activity of enhancers. While the original protocol facilitated the discovery of previously unannotated or undetectable RNA classes, including enhancers, that method requires a multi-day workflow and a large amount of input material (10-30 million cells). Given the unstable nature of enhancers, our lab in close collaboration with the Lis Lab through a joint-postdoctoral fellow, streamlined the protocol to significantly reduce the experimental handling time (~14 hours) and permit the use of limited cell numbers (as low as 100-500K) while retaining the high sensitivity and specificity of the assay. In addition, this faster and more versatile assay allows the use of a variety of biospecimens. To date, we have extensive experience in generating PRO-cap libraries. For example, we have generated high-quality PRO-cap libraries using different human cell types from commercial sources and primary and organoid cultures, solid tissues from cadaveric or surgical biopsies, and liquid biopsies such as blood and their FACS-sorted cell fractions. Likewise, our group frequently utilizes PRO-seq (Precision Run-On Sequencing; developed by the Lis Lab) to map the genome-wide positions and density of all active transcriptionally-engaged RNA polymerases.
Structural proteomics using deep learning methods
We develop a deep-learning-based ensemble learning pipeline to generate accurate partner-specific protein-protein interface prediction with significant improvement in both quality and coverage for protein interactions. We integrate experimental structures and homology models to create multi-scale 3D structural interactomes, combined with disease mutations and functional annotations in a dynamic web server for genome-wide functional genomics studies.Relative Publication: Meyer et al. Nature Methods, 2018 Weirbowski et al. Nature Methods, 2022;
Genomics and epigenomics data analysis using deep learning
We use Graph-Attention Neural Networks to model enhancer-promoter connections and learn regulatory relationships from genomics and epigenomics assays such as PRO-cap and HiC. Our deep learning tools, including EnhancerNet and PRO-cap deconvolution, unravel the functional and architectural aspects of enhancer elements, enabling accurate identification of enhancer units with their boundaries across the genome. This approach provides unique and comprehensive perspectives on endogenous enhancer activity and regulatory rules between enhancers and promoters.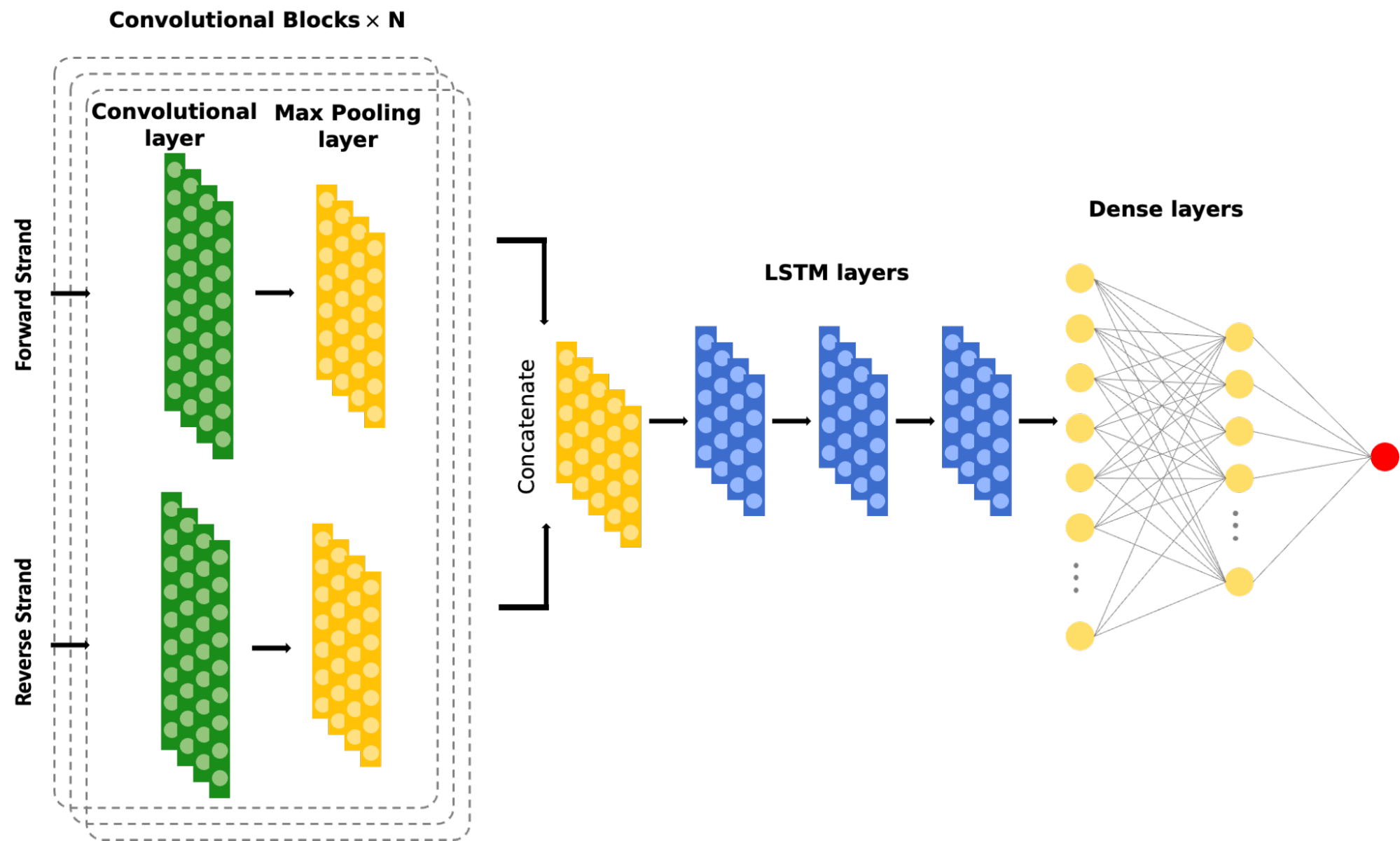
Enhancers are crucial in regulating gene expression dynamics in a cell-type-specific manner. However, accurately identifying functional enhancer units with their boundaries across the genome is challenging. To address this, we are developing a suite of deep learning tools that can unravel the genome-wide architectural and functional aspects of enhancer elements. The first tool in our library is EnhancerNet, which uses composite Convolutional Neural Networks (CNN) and Long Short-Term Memory (LSTM) models followed by fully connected layers to predict enhancer elements at a base-pair resolution. This model dissects signals from PRO-cap assays and understands the signal shape features.
Prioritization of coding and noncoding variants genome-wide
Missense variants are important for diagnosing genetic disorders and predicting disease risk. However, given the uncertainty of functional effects, a large proportion of missense variants reported from genetic testing are labeled as variants of uncertain significance. Previous studies have shown that protein sequence and local structure contain rich information for inferring variant disease impact. Recent advances of graph neural networks (GNNs) offer powerful tools to extract information and make predictions using data with complex relationships. We would like to utilize graph neural networks to predict functional effects of variants by leveraging different connectivities and features corresponding to protein primary sequence, 3D structure, co-evolution strength, etc. We hope that the well-trained model would be able to learn latent representations of protein functional sites in terms of biophysical and spatial properties, and thus provide highly reliable prediction of variant functional effects.Relative Publication: Chen et al. Nature Genetics, 2018

- In our lab, we employ advanced network analysis techniques to identify functional modules within cells that play crucial roles in the development of human diseases. By analyzing large-scale molecular interaction data, such as protein-protein, enhancer-promoter, protein-DNA, and gene co-expression networks, we aim to uncover the intricate relationships between various cellular components and their involvement in disease processes. Our approach involves the detection of highly interconnected subnetworks or modules that are associated with specific biological functions, cellular processes, or pathways. These functional modules can provide valuable insights into the molecular mechanisms underlying disease onset and progression, helping to identify potential therapeutic targets and enhance our understanding of complex biological systems. By harnessing the power of network analysis, we strive to advance our knowledge of cellular organization and function in the context of human diseases, ultimately contributing to the development of novel diagnostic and therapeutic strategies.
Relative Publication: Zhang et al. 2023 (In Press)